The Evolution of Artificial Intelligence: From Sci-Fi Dreams to Everyday Reality
There was a time, still within living memory, when the term “artificial intelligence” conjured images of distant sci-fi futures where automata lived alongside humans—sometimes in utopian harmony and helpful assistance, other times as dystopian overlords in tales warning of humanity’s overreach in technological development. As of 2024, however, the everyday reality of artificial intelligence has taken on a more mundane, embedded, and infrastructural significance.
What we now refer to as AI is applied across various domains, including scientific and medical research, industrial automation, crime mapping, facial recognition, personal assistants, customer service, and—most relevant to this discussion—social media platforms.
While discussions about AI are ubiquitous, our actual encounters with AI often occur in the background of everyday interactions.
In these contexts, “artificial intelligence” is more accurately described as machine learning, where large datasets, unprecedented storage capacity, and new algorithmic analysis techniques converge to facilitate pattern recognition, classification, transcription, automated decision-making, and prediction.
Myths and Misinformation: Public Perceptions of Machine Learning
Although the process of machine learning is not particularly novel, there remains significant misinformation and perceptual divides in how the public views AI. Scholars have long noted the myths surrounding AI and the “folk theories” of algorithms, and recent surveys indicate growing public apprehension regarding AI.
Contemporary machine learning is more akin to a crystal ball than a thinking machine.
Perhaps most troubling are findings from some surveys showing that portions of the population believe AI to be sentient and self-aware—a significant departure from the reality of what machine learning entails.
These misconceptions are likely fueled by the relentless hype generated by big tech companies, even as cracks begin to appear in their narratives. AI is often touted as “turbocharging productivity,” yet this is juxtaposed with months of mass layoffs in the tech industry.
AI also necessitates massive increases in power consumption in an era of climate change and strained power grids. Furthermore, predictions suggest that the increased adoption of AI could widen the income gap between capital and labor. Perhaps the greatest concern for profit-driven entities is the projection that massive investments in AI may ultimately prove unprofitable.
The Opaque Reality: Unpacking the Hidden Mechanisms of AI
In a sense, what we have come to know as AI is both omnipresent and opaque. While discussions about AI are ubiquitous, our actual encounters with AI often occur in the background of everyday interactions. The mechanisms of these background machine learning processes are intentionally obscured; the algorithms driving various forms of machine learning are closely guarded proprietary secrets.
This creates a rather disempowering yet familiar situation: “buy the hype, use the technology, give us your data, and don’t look under the hood.” Instead of accepting this unknowable “black box” of artificial intelligence, researchers have devised strategies to pry open the mystery and begin to demystify the algorithms that structure many of our interactions.
Recommendation algorithms shift based on use practices due to user activity and data.
Currently, large language models like OpenAI’s ChatGPT draw considerable attention; however, it is crucial to assess other forms of machine learning that, though infrastructural and invisible, shape everyday interactions, often in potentially harmful ways. Inspired by recent scholarship, I set out to better understand the embedded artificial intelligence of Facebook and TikTok.
To this end, I examined recent transparency initiatives, official statements by platform representatives, and pieced together available information on how AI is integrated into these two ubiquitous platforms, with a particular focus on how AI is promoted by big tech as a solution to the circulation of problematic and dangerous content, as well as disinformation and misinformation.
The Complexity of Defining Artificial Intelligence
There are several persistent challenges when it comes to critically assessing embedded AI algorithms.
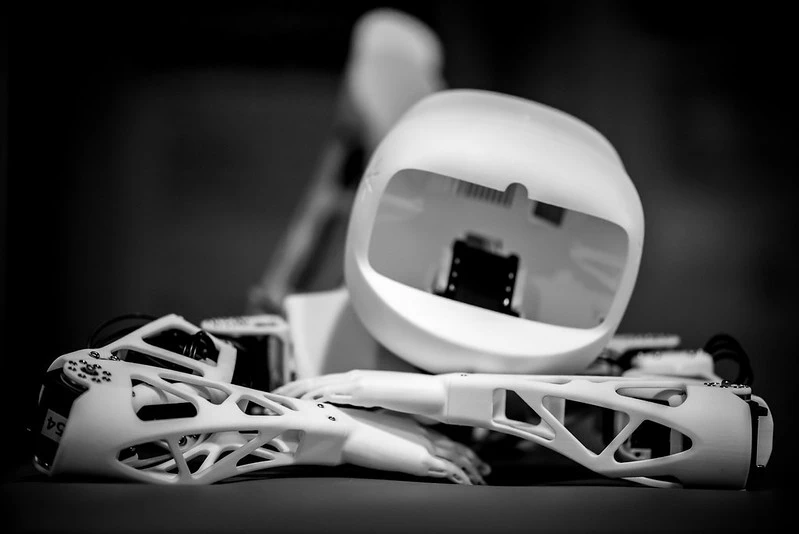
First is the cloudiness that accompanies the question of what “AI” entails. A look back at the historical origins of artificial intelligence in the 1950s demonstrates the changing theoretical and technical underpinnings of AI.
AI is “sociotechnical,” insofar as it is deeply intertwined with the contexts and situations in which it is deployed.
That is, AI is driven not only by computational structures (and limitations), but also by a theory of what constitutes “intelligence” in the first place.
The machine learning algorithms underpinning social media platforms are quite different from the symbolic AI of the mid-1950s, both in design and philosophy. At the risk of oversimplification, classical AI attempted to replicate the mind through the manipulation of human-readable symbols according to underlying rules.
These models have largely been replaced with a more predictive and analytical notion of intelligence comprised of layered algorithms, huge datasets, and substantial processing power. In other words, contemporary machine learning is more akin to a crystal ball than a thinking machine.
In some everyday encounters, like on social media, infrastructural versions of machine learning AI are often referred to as “the algorithm.” AI is, in this way, also relatively polysemic in how it is conceptualized and discussed.
The Proprietary Nature and Sociotechnical Reality of AI
Second, AI models are often heavily protected by companies who want to maintain an edge over competitors. For illustration, while nearly all streaming platforms employ predictive analytics to recommend content, the kinds of datasets used in training these models, as well as the parameters algorithms weight, vary by platform.
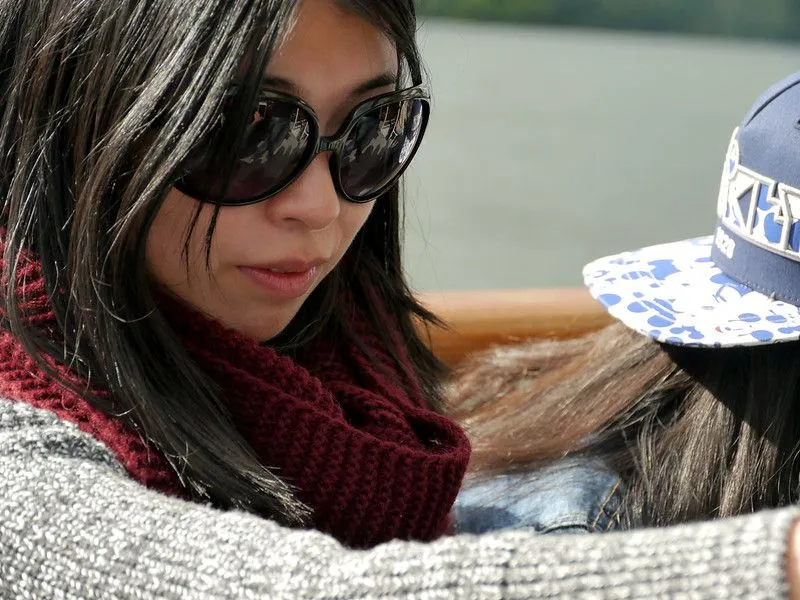
These proprietary algorithms explain part of why TikTok is so popular while YouTube Shorts isn’t, or why people prefer Google to Bing despite the similarity in function of such platforms.
It’s more essential than ever to interrogate the discursive and material apparatuses of embedded machine learning models.
Third, there exist assumptions about algorithmic stability. That is, while big tech and computer scientists are quick to define algorithms as established computational techniques, the reality of embedded data-driven AI processes is far more shifting and entangled with the social via datasets.
Stated differently, AI is “sociotechnical,” insofar as it is deeply intertwined with the contexts and situations in which it is deployed. Recommendation algorithms, for instance, shift based on use practices due to user activity and data. This fact allows for experimentation, tinkering, and observation of algorithms by researchers.
Experimental Approaches to Understanding AI
In summation, assessing how AI functions is a complex problem: the term AI is used liberally to refer to a variety of technical processes driven by philosophies of the mind; AI is historically constrained; AI models are proprietary secrets; and AI isn’t inherently a stable technical object, but instead sociotechnical. Consequently, pulling at the threads of how embedded AI functions is thorny, but not impossible. To do so requires not only assessments of the technical function of AI but also the context of use, as well as how AI is framed in discourses by major power structures.
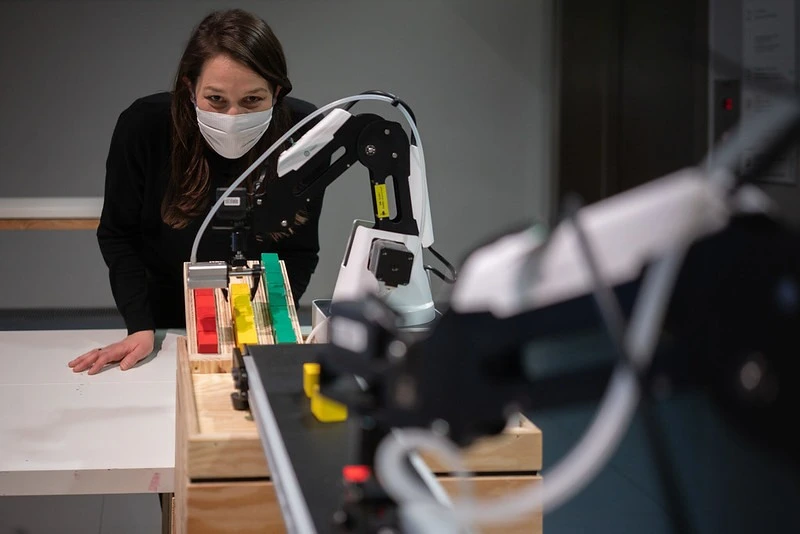
Scholars have recently turned to more experimental strategies to examine the complexity of embedded algorithmic processes. This includes considering the relational nature of algorithms, how humans and machines are intertwined in complex material, political, economic, and organizational relations, and how algorithms are part of broad patterns of cultural meaning and practice that can be empirically engaged with. Specifically, I endeavored to access the embedded machine learning AI of Facebook and TikTok as a “material-discursive apparatus.”
This required considering official discourses surrounding these platforms’ AI techniques as a structure of power in terms of what is seeable, sayable, and knowable about AI, along with information about how AI is embedded in these platforms via algorithms, datasets, users, platforms, infrastructures, moderators, etc. As such, the use of AI as part of Facebook and TikTok demonstrates that AI does not exist in isolation as a stable technical object but is better understood as an ongoing process reliant on strategies of acceptance via discursive techniques and the changing material arrangements of everyday embeddedness.
The Love-Hate Relationship with Facebook and TikTok
Facebook and TikTok are emblematic of our love-hate societal relationship with platforms. The potential of these social media nexuses for bringing people together and fostering new connectivity is often rightfully juxtaposed with well-earned criticism and an aura of infamy.
Facebook has been popular for two decades, while TikTok’s meteoric rise is relatively recent. Facebook’s demographics now trend older, whereas TikTok is emblematic of younger generations’ digital interactions. Yet both platforms share in controversy.
Facebook is at the epicenter of debates regarding bias, the spread of misinformation and disinformation, political manipulation, and unethical data-sharing practices. TikTok similarly has come under fire and even faced bans in certain countries for its perceived relationship with the Chinese Communist Party and its data collection practices.
Transparency and the Realities of AI Moderation
It comes as little surprise that in response to this negative press, Facebook and TikTok each launched transparency initiatives providing details on how the platforms’ embedded machine learning algorithms function.
These initiatives, along with statements and commentary by platform representatives, as well as additional information on how algorithms function on each platform, provide a rare look at how these platforms articulate their use of machine learning, which often runs counter to some of the realities of these sociotechnical processes.
There is an intentional fuzziness around AI that benefits tech companies.
For example, Facebook has invested heavily in AI, including the release of open-AI tools and touting their Rosetta AI system as key to moderating problematic content that, left unchecked, can easily circulate on the platform. Additional details on how Facebook uses AI to moderate content shed some light on how the process has changed over time.
Posts that potentially contain harmful content are flagged—either by users or machine learning filters—then a human moderator sorts through the flagged posts for removal. Moreover, while this process functioned chronologically in the past, in that posts were dealt with in the order they were reported, Facebook’s newer algorithms prioritize what moderators should view first based on criteria of virality, severity, and the likelihood of a post breaking platform rules.
The Limits of Facebook’s AI
Perhaps most interesting of all are the limitations of Facebook’s use of AI. Facebook’s machine learning filters analyze posts via “whole post integrity embeddings” (WPIE), which judge various elements of a given post. But breaking down post elements into discrete data points that are compared to previous cases means Facebook’s AI is unable to determine what the images, captions, and relationships to the poster reveal.
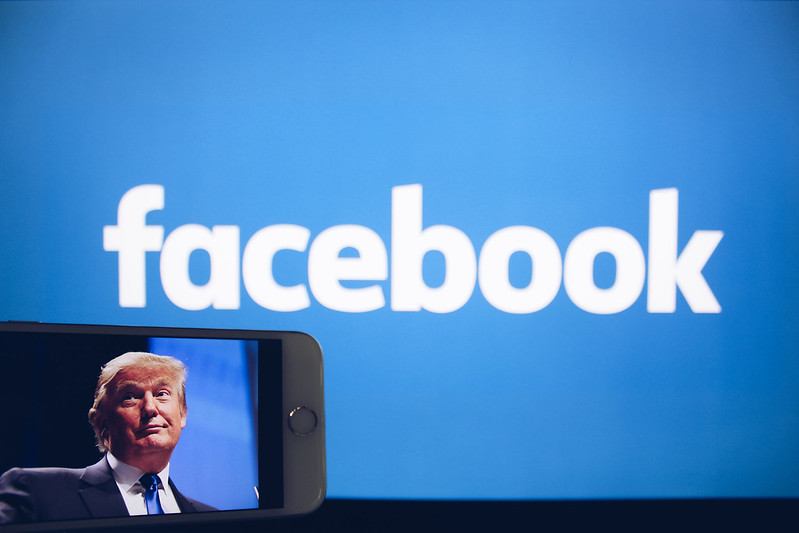
For example, a photo of Rice Krispies squares labeled “special treats” could be shorthand for THC edibles or merely delicious baked goods. It’s up to a human moderator to apply critical reasoning to decipher whether flagged content is truly an issue worthy of removal.
This example is relatively trivial; yet, these human moderators, made intentionally invisible by platforms, are subject to viewing some of the most horrendous content posted to the web, often at low wages and without mental health support.
TikTok’s Algorithm: Successes and Shortcomings
Investigation into TikTok’s machine learning AI raises similar issues. Due to controversies surrounding the platform’s use of data, TikTok has been somewhat forthcoming about its algorithm.
The company notes that recommendations are based on factors such as user interactions (videos that are liked or shared, followed accounts, comments posted), video information (captions, sounds, hashtags), and device and account settings (language preference, device type, country settings).
There has been speculation that TikTok’s AI can recognize images in uploaded videos for categorization, recommendation, and moderation. However, it appears that, like YouTube and Facebook, TikTok’s machine learning models primarily rely on metadata such as descriptions, tags, time, and location of video uploads. In other words, TikTok uses AI for recommendation, selection, and personalization, much like other platforms. It merely does so more successfully.
The Illusion of AI Independence
One notable aspect of TikTok is that the platform emphasizes how the company’s proprietary algorithm interrupts repetitive patterns and duplicate content to diversify recommendations and “burst filter bubbles.”
Research and experimentation into the spread of radicalized and controversial political content circulating on the platform have found that the reality of TikTok’s bubble-bursting AI and content moderation is lacking. Leaked documents regarding guidelines for human moderators revealed that TikTok aimed to remove content from users who have, or appear to have, an “abnormal body shape.”
This includes users described as “chubby,” “obese or too thin,” having “ugly facial looks,” or “facial deformities.” The guidelines also targeted “senior people with too many wrinkles” and those filming videos in environments considered “shabby,” “dilapidated,” “slums,” or “rural fields.” The platform was also willing to remove political content to appease certain governments.
All in all, machine learning AI is deployed differently in Facebook and TikTok, even if these models often function toward similar ends. Despite the promises of AI, accessed via discursive positioning by platform statements and representatives, the material realities of how machine learning functions often fail to live up to the hype.
No matter the platform, human moderation (along with human-created data, training, and programming) is inexorably intertwined with AI. And perhaps most significant of all, it would be quite the exaggeration to consider data-driven algorithmic models “intelligent” in the sense that there is any kind of critical thought or even particularly impressive reliability.
The Future of Everyday AI
Hype is hard to ignore. It’s admittedly fun to imagine the possibilities of an AI-driven future, in which algorithmic models replace tedious and mundane tasks, solve complex issues, and free humans from drudgery. Tech companies would have the public believe our AI-powered destiny is right around the corner, but the reality is something far messier.
Contemporary AI can be impressive and even useful at times, but it is also a far cry from human intelligence. These models are adept at solving certain kinds of close-ended problems with clear goals—Deep Blue rivaled chess masters decades ago. However, tasks like moderating hate speech on platforms aren’t games with limited permutations; they are complex problems involving historical and contemporary knowledge, judgment, context, and critical thought. As of now, these are among the many problems that machine learning can’t easily solve, despite narratives to the contrary by big tech.
Amidst what many consider an AI bubble (perhaps one showing signs of popping), it’s more essential than ever to interrogate the discursive and material apparatuses of embedded machine learning models. As previously mentioned, there is an intentional fuzziness around AI that benefits tech companies. This opacity contributes to messy discussions in which terminology like algorithms, machine learning, AI, and big data are used interchangeably to describe infrastructural and embedded computerized processes.
Most concerningly, the impenetrability of AI leads to bold claims, confusion, and a general overreliance on the notion that “technology will solve all.” For instance, recent comments by Bill Gates suggest that AI will solve the issue of the energy demands of the data centers powering AI. Or, as briefly recapped here, the claims of successful AI moderation by Facebook and TikTok, when the actual outcomes are far more mixed.
What we’re left with is an intentionally obfuscated situation—one that necessitates going beyond hype cycles to look under the hood of embedded machine learning AI. Contemporary research offers novel strategies for considering how algorithmic processes are both social and technical, along with new ways of accessing the shifting nature of embedded machine learning. The ongoing task for researchers is to resist positioning AI as a stable technical entity or buying into narratives of unilateral AI benefits. Instead, they should examine the ongoing material-discursive arrangements of what AI is, what AI is becoming, and how AI is integrated into everyday practices.